Chapter 3 Quick start
3.1 About
- Although with bright prospects in Pan-disease analysis, GSClassifier was primarily developed for clinic-friendly immune subtypes of gastric cancer (GC). Currently, only
PAD
subtypes andPADi
for GC were supported. We would try to support more cancer types in the future as possible. More details in Plans in the future section. - Gibbs'
PanCancer immune subtypes
based on five gene signatures (485 genes) could also be called inGSClassifier
, with a pre-trained model from the ImmuneSubtypeClassifier package. If you use their jobs, please cite these papers. - Particularly, all normal tissues should be eliminated before subtype identificaiton for cancer research.
3.2 Package
# Install "devtools" package
if (!requireNamespace("devtools", quietly = TRUE))
install.packages("devtools")
# Install dependencies
if (!requireNamespace("luckyBase", quietly = TRUE))
devtools::install_github("huangwb8/luckyBase")
# Install the "GSClassifier" package
if (!requireNamespace("GSClassifier", quietly = TRUE))
devtools::install_github("huangwb8/GSClassifier")
# Load needed packages
library(GSClassifier)
# Loading required package: luckyBase
3.3 Data
To lower the learning cost of GSClassifier
, we provide some test data:
Explore the testData
:
3.4 PAD
3.4.1 The work flow of PAD exploration
The basic process of PAD exploration was summarized in Figure 3.1
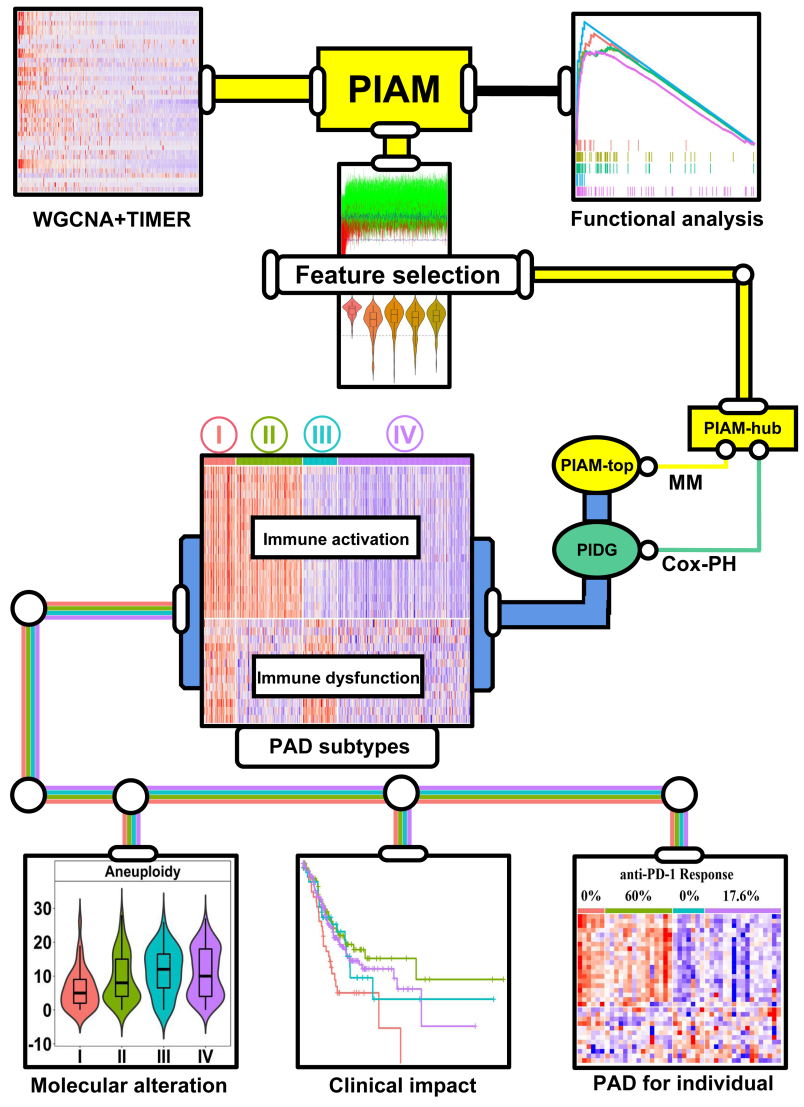
Figure 3.1: The process of PAD subtypes establishment
With WGCNA method and TIMER datasets, Pan-Immune Activation Module (PIAM) was identified as a GEP representing co-infiltration of immune cells in the tumor microenvironment. Functional analysis such as GSEA was done for the exploration of PIAM functions.
With feature selection based on Boruta algorithm, missing value control (without missing value in over 80% of GC datasets we used), we retained 101 genes and named as “PIAM-hub”.
Pan-Immune Dysfunction Genes (PIDG) were explored based on “PIAM-hub” via a strategy similar to the computational framework of the Tumor Immune Dysfunction and Exclusion (TIDE) database [20]. Finally, 13 PIDGs were selected for downstream analysis as they were further validated in 2 or more external GC cohorts.
To further reduce PIAM-hub for downstream modeling, genes with Mean of Correlation with Eigengene (MCE) ≥0.8 were selected and termed as “PIAM-top” subset (n=19).
“PIAM-top” and PIDG, two curated tiny GEPs, were applied to establish Pan-immune Activation and Dysfunction (PAD) subtypes (PAD-I, PIAMhighPIDGhigh; PAD-II, PIAMhighPIDGlow; PAD-III, PIAMlowPIDGhigh; and PAD-IV, PIAMlowPIDGlow) in independent GC cohorts.
Molecular alteration and patient survival across PAD subtypes were analyzed to figure out its biological and clinical impact. Also, a GSClassifier model called “PAD for individual” (PADi) was established for personalized subtype identification for immune checkpoint inhibitors response prediction in GC (More details in Online Section/PDF).
3.4.2 Preparation of the test data
Load phenotype data:
design <- testData$PanSTAD_phenotype_part
table(design$Dataset)
#
# GSE13861 GSE13911 GSE15459 GSE22377 GSE26899 GSE26901 GSE29272 GSE51105
# 65 39 192 43 96 108 134 94
# GSE54129 GSE57303 GSE62254 GSE65801 GSE84437 TCGA-STAD
# 111 70 300 32 433 372
Load target sample IDs in GSE54129
cohort:
target_ID <- design$ID[design$Dataset %in% 'GSE54129']
expr <- testData$PanSTAD_expr_part[,target_ID]
head(expr[,1:10])
# GSM1308413 GSM1308414 GSM1308415 GSM1308416 GSM1308417
# ENSG00000122122 7.888349 7.623663 6.873493 6.961102 7.150572
# ENSG00000117091 7.051760 6.217445 5.651839 5.830996 5.908532
# ENSG00000163219 6.056472 5.681844 5.411533 5.652684 5.555147
# ENSG00000136167 9.322191 8.765794 8.502315 8.838166 8.845952
# ENSG00000005844 7.119594 6.023631 5.400999 6.172863 6.059838
# ENSG00000123338 7.204051 6.925328 6.259809 6.610681 6.595882
# GSM1308418 GSM1308419 GSM1308420 GSM1308421 GSM1308422
# ENSG00000122122 7.871423 6.953329 8.334037 6.764335 6.522554
# ENSG00000117091 6.526917 5.646446 6.617520 5.637693 5.742848
# ENSG00000163219 5.962885 5.361763 5.975842 5.330428 5.172705
# ENSG00000136167 9.366074 8.675718 9.118517 8.614068 8.114096
# ENSG00000005844 6.523530 6.129181 7.331588 5.547059 5.867118
# ENSG00000123338 6.699790 6.935390 7.050288 6.536710 6.200269
3.4.3 Unsupervised clustering
res_pad <- PAD(
expr = expr,
cluster.method = "ward.D2",
extra.annot = NULL,
plot.title = 'PAD test',
subtype = "PAD.train_20220916",
verbose = T
)
# Use default PIAM...
# Use default PIDG...
# Gene match: 100%.
# Done!
3.4.4 Of note
It’s strongly recommended that the gene type of
expr
should be always the same, such as ENSEMBL genes (ENSG00000111640 for GAPDH, for example).PAD
function is only for datasets with lots of samples for its classification depends on population-based unsupervised clustering.PAD
is population-dependent and non-personalized.Beta characteristics: You could try random forest classification based on the
randomForest
package or methods instats::hclust
.
3.5 PADi
In
GSClassifier
,PADi
is a pre-trained out-of-the-box model for GC personalized PAD subtypes calling.During the subtype calling, the gene rank relations based on individuals instead of the relative values across samples would be used. Thus, you don’t have to do batch normalization even though the data (the
X
input) come from multiple cohorts or platforms.More limitations were discussed in our paper that you had better know.
In this section, we would show how to use PADi
function series: PADi
, callEnsemble
, and parCallEnsemble
functions.
3.5.1 Preparation of the test data
X <- testData$Kim2018_3
head(X)
# PB-16-002 PB-16-003 PB-16-004
# ENSG00000121410 0.07075272 -2.08976724 -1.43569557
# ENSG00000148584 -1.49631022 -0.23917056 0.94827471
# ENSG00000175899 -0.77315329 0.52163146 0.91264015
# ENSG00000166535 -0.28860715 -0.45964255 -0.38401295
# ENSG00000256069 -0.25034243 0.06863867 0.14429081
# ENSG00000184389 0.08215945 -0.05966481 0.04937924
3.5.2 Use a specific function called PADi
Very simple, just:
Check the result:
head(res_padi)
# SampleIDs BestCall BestCall_Max 1 2 3 4
# 1 PB-16-002 4 4 0.023372779 0.02631794 0.04864784 0.3336484
# 2 PB-16-003 4 4 0.007271698 0.08650742 0.01974812 0.9530730
# 3 PB-16-004 4 4 0.011559768 0.02922151 0.09018894 0.8649045
Actually, PADi
is exactly based on a general function called callEnsemble
.
3.5.3 Use the callEnsemble
function
Also simple, just:
res_padi <- callEnsemble(
X = X,
ens = NULL,
geneAnnotation = NULL,
geneSet = NULL,
scaller = NULL,
geneid = "ensembl",
matchmode = 'fix',
subtype = "PAD.train_20220916",
verbose = F
)
Check the result:
3.5.4 Parallel strategy for PADi
Sometimes, the number of patients for subtype callings could be huge (hundreds or even tens of thousands). Thus, parallel computing (Windows or Linux pass; not tested in Mac or other OS) was also developed in the current version of the
GSClassifier
package.The parameter
numCores
was used to control the No. of CPU for computing (which depends on your CPU capacity).
# No run for the tiny test data. With errors.
# Method 1:
res_padi <- PADi(X = X, verbose = F, numCores = 4)
# Method 2:
res_padi <- parCallEnsemble(
X = X,
ens = NULL,
geneAnnotation = NULL,
geneSet = NULL,
scaller = NULL,
geneids = 'ensembl',
matchmode = 'fix',
subtype = 'PAD.train_20220916',
verbose = T,
numCores = 4)
3.5.5 Single sample subtype calling
In clinical practice, the single sample subtype calling might be one of the most common scenarios and is also supported by functions of the PADi
series.
Supposed that there is a GC patient, its information should be:
X_ind <- X[,1]; names(X_ind) <- rownames(X)
head(X_ind)
# ENSG00000121410 ENSG00000148584 ENSG00000175899 ENSG00000166535 ENSG00000256069
# 0.07075272 -1.49631022 -0.77315329 -0.28860715 -0.25034243
# ENSG00000184389
# 0.08215945
Or it can also be another format:
X_ind <- as.matrix(X[,1]); rownames(X_ind) <- rownames(X)
head(X_ind)
# [,1]
# ENSG00000121410 0.07075272
# ENSG00000148584 -1.49631022
# ENSG00000175899 -0.77315329
# ENSG00000166535 -0.28860715
# ENSG00000256069 -0.25034243
# ENSG00000184389 0.08215945
Similar to multiples sample calling, just:
check the result:
head(res_padi)
# SampleIDs BestCall BestCall_Max 1 2 3 4
# 1 target 4 4 0.02337278 0.02631794 0.04864784 0.3336484
Similarly, there is alternative choice:
res_padi <- callEnsemble(
X = X_ind,
ens = NULL,
geneAnnotation = NULL,
geneSet = NULL,
scaller = NULL,
geneid = "ensembl",
matchmode = 'fix',
subtype = "PAD.train_20220916",
verbose = F
)
Check the result:
3.5.6 Of note
In the results of
PADi
, two types of subtypes (BestCall
andBestCall_Max
) were integrated.BestCall
was predicted via an xgboost model based on prior knowledge ofPAD
subtypes and the possibility matrix (columns 4 to 7 of four-subtype calling, for example), whileBestCall_Max
was predicted via maximum strategy. Empirically,BestCall
seemed to be a better choice.PADi
is individual-dependent and personalized, which means that the result of subtype calling would not be influenced by the data of others.
3.6 Use external models from luckyModel package
In the future, there might be lots of models available as a resource of GSClassifier
, such as luckyModel. Here we show how luckyModel
support GSClassifier
.
First, install and load luckyModel
:
# Install luckyModel
if (!requireNamespace("luckyModel", quietly = TRUE))
devtools::install_github("huangwb8/luckyModel")
library(luckyModel)
Check projects supported in current luckyModel
:
Check available models in the project:
list_model(project='GSClassifier')
# Available models in GSClassifier:
# *Gibbs_PanCancerImmuneSubtype_v20190731
# *HWB_PAD_v20200110
# *HWB_PAD_v20220916
Here, HWB_PAD_v20200110
is a standard name of PADi
. They are the same.
Taking PADi
as an example, we here show how to use an external model from luckyModel
. First, load a model:
model <- lucky_model(project = 'GSClassifier',
developer='HWB',
model = 'PAD',
version = 'v20200110')
Then, check the gene id type:
model$geneSet
# $PIAM
# [1] "ENSG00000122122" "ENSG00000117091" "ENSG00000163219" "ENSG00000136167"
# [5] "ENSG00000005844" "ENSG00000123338" "ENSG00000102879" "ENSG00000010671"
# [9] "ENSG00000185862" "ENSG00000104814" "ENSG00000134516" "ENSG00000100055"
# [13] "ENSG00000082074" "ENSG00000113263" "ENSG00000153283" "ENSG00000198821"
# [17] "ENSG00000185811" "ENSG00000117090" "ENSG00000171608"
#
# $PIDG
# [1] "ENSG00000116667" "ENSG00000107771" "ENSG00000196782" "ENSG00000271447"
# [5] "ENSG00000173517" "ENSG00000134686" "ENSG00000100614" "ENSG00000134247"
# [9] "ENSG00000109686" "ENSG00000197321" "ENSG00000179981" "ENSG00000187189"
# [13] "ENSG00000140836"
The model should use ensembl
as the value of geneid
parameter in callEnsemble
series.
Next, you can use the model like:
res_padi <- callEnsemble(
X = X,
ens = model$ens$Model,
geneAnnotation = model$geneAnnotation,
geneSet = model$geneSet,
scaller = model$scaller$Model,
geneid = "ensembl",
matchmode = 'fix',
subtype = NULL,
verbose = F
)
Or just:
res_padi <- callEnsemble(
X,
ens = NULL,
geneAnnotation = NULL,
geneSet = NULL,
scaller = NULL,
geneid = "ensembl",
matchmode = 'fix',
subtype = model,
verbose = F
)
They are exactly the same.
Finally, check the result:
3.7 PanCancer immune subtypes
GSClassifier
could also call the PanCancer immune subtypes
of Gibbs’ [2].
First, see data available in current GSClassifier
:
GSClassifier_Data()
# Available data:
# Usage example:
# ImmuneSubtype.rds
# PAD.train_20200110.rds
# PAD.train_20220916.rds
# PAD <- readRDS(system.file("extdata", "PAD.train_20200110.rds", package = "GSClassifier"))
# ImmuneSubtype <- readRDS(system.file("extdata", "ImmuneSubtype.rds", package = "GSClassifier"))
Let’s use our test data to do this:
X <- testData$Kim2018_3
symbol <- convert(rownames(X))
rownames(X) <- symbol
X <- X[!is.na(symbol),]
dim(X)
# [1] 19118 3
Have a check
head(X)
# PB-16-002 PB-16-003 PB-16-004
# A1BG 0.07075272 -2.08976724 -1.43569557
# A1CF -1.49631022 -0.23917056 0.94827471
# A2M -0.77315329 0.52163146 0.91264015
# A2ML1 -0.28860715 -0.45964255 -0.38401295
# RP11-118B22.6 -0.25034243 0.06863867 0.14429081
# A3GALT2 0.08215945 -0.05966481 0.04937924
PanCan Immune Subtype callings:
res_pis <- callEnsemble(
X = X,
ens = NULL,
geneAnnotation = NULL,
geneSet = NULL,
scaller = NULL,
geneid = "symbol",
matchmode = 'free',
subtype = "ImmuneSubtype",
verbose = F
)
Check the result:
head(res_pis)
# SampleIDs BestCall BestCall_Max 1 2 3
# 1 PB-16-002 2 2 1.023672e-03 0.561300665 7.031194e-06
# 2 PB-16-003 4 4 4.206522e-07 0.018167170 1.082299e-04
# 3 PB-16-004 3 3 2.705873e-06 0.001126488 3.505213e-01
# 4 5 6
# 1 0.2488089278 0.009215117 0.003069826
# 2 0.4748829454 0.002282911 0.002286367
# 3 0.0002505876 0.007858477 0.007010047
Also, you can try to use luckyModel
:
pci <- lucky_model(
project = "GSClassifier",
model = "PanCancerImmuneSubtype",
developer = "Gibbs",
version = "v20190731"
)
PanCan Immune Subtype callings:
res_pis <- callEnsemble(
X = X,
ens = NULL,
geneAnnotation = NULL,
geneSet = NULL,
scaller = NULL,
geneid = "symbol",
matchmode = 'free',
subtype = pci,
verbose = F
)
Finally, we take a look at the PanCancer immune subtypes
model:
ImmuneSubtype <- readRDS(system.file("extdata", "ImmuneSubtype.rds", package = "GSClassifier"))
names(ImmuneSubtype)
# [1] "ens" "scaller" "geneAnnotation" "geneSet"
Its gene annotation:
head(ImmuneSubtype$geneAnnotation)
# SYMBOL ENTREZID ENSEMBL
# 235 ACTL6A 86 ENSG00000136518
# 294 ADAM9 8754 ENSG00000168615
# 305 ADAMTS1 9510 ENSG00000154734
# 322 ADAR 103 ENSG00000160710
# 340 ADCY7 113 ENSG00000121281
# 479 AIMP2 7965 ENSG00000106305
Its gene sets:
ImmuneSubtype$geneSet
# $LIexpression_score
# [1] "CCL5" "CD19" "CD37" "CD3D" "CD3E" "CD3G" "CD3Z" "CD79A" "CD79B"
# [10] "CD8A" "CD8B1" "IGHG3" "IGJ" "IGLC1" "CD14" "LCK" "LTB" "MS4A1"
#
# $CSF1_response
# [1] "CORO1A" "MNDA" "CCRL2" "SLC7A7" "HLA-DMA" "FYB"
# [7] "RNASE6" "TLR2" "CTSC" "LILRB4" "PSCDBP" "CTSS"
# [13] "RASSF4" "MSN" "CYBB" "LAPTM5" "DOCK2" "FCGR1A"
# [19] "EVI2B" "ADCY7" "CD48" "ARHGAP15" "ARRB2" "SYK"
# [25] "BTK" "TNFAIP3" "FCGR2A" "VSIG4" "FPRL2" "IL10RA"
# [31] "IFI16" "ITGB2" "IL7R" "TBXAS1" "FMNL1" "FLI1"
# [37] "RASSF2" "LYZ" "CD163" "CD97" "CCL2" "FCGR2B"
# [43] "MERTK" "CD84" "CD53" "CD86" "HMHA1" "CTSL"
# [49] "EVI2A" "TNFRSF1B" "CXCR4" "LCP1" "SAMHD1" "CPVL"
# [55] "HLA-DRB1" "C13orf18" "GIMAP4" "SAMSN1" "PLCG2" "OSBPL3"
# [61] "CD8A" "RUNX3" "FCGR3A" "AMPD3" "MYO1F" "CECR1"
# [67] "LYN" "MPP1" "LRMP" "FGL2" "NCKAP1L" "HCLS1"
# [73] "SELL" "CASP1" "SELPLG" "CD33" "GPNMB" "NCF2"
# [79] "FNBP1" "IL18" "B2M" "SP140" "FCER1G" "LCP2"
# [85] "LY86" "LAIR1" "IFI30" "TNFSF13B" "LST1" "FGR"
# [91] "NPL" "PLEK" "CCL5" "PTPRC" "GNPTAB" "SLC1A3"
# [97] "HCK" "NPC2" "C3AR1" "PIK3CG" "DAPK1" "ALOX5AP"
# [103] "CSF1R" "CUGBP2" "APOE" "APOC1" "CD52" "LHFPL2"
# [109] "C1orf54" "IKZF1" "SH2B3" "WIPF1"
#
# $Module3_IFN_score
# [1] "IFI44" "IFI44L" "DDX58" "IFI6" "IFI27" "IFIT2" "IFIT1" "IFIT3"
# [9] "CXCL10" "MX1" "OAS1" "OAS2" "OAS3" "HERC5" "SAMD9" "HERC6"
# [17] "DDX60" "RTP4" "IFIH1" "STAT1" "TAP1" "OASL" "RSAD2" "ISG15"
#
# $TGFB_score_21050467
# [1] "MMP3" "MARCKSL1" "IGF2R" "LAMB1" "SPARC" "FN1"
# [7] "ITGA4" "SMO" "MMP19" "ITGB8" "ITGA5" "NID1"
# [13] "TIMP1" "SEMA3F" "RHOQ" "CTNNB1" "MMP2" "SERPINE1"
# [19] "EPHB2" "COL16A1" "EPHA2" "TNC" "JUP" "ITGA3"
# [25] "TCF7L2" "COL3A1" "CDH6" "WNT2B" "ADAM9" "DSP"
# [31] "HSPG2" "ARHGAP1" "ITGB5" "IGFBP5" "ARHGDIA" "LRP1"
# [37] "IGFBP2" "CTNNA1" "LRRC17" "MMP14" "NEO1" "EFNA5"
# [43] "ITGB3" "EPHB3" "CD44" "IGFBP4" "TNFRSF1A" "RAC1"
# [49] "PXN" "PLAT" "COL8A1" "WNT8B" "IGFBP3" "RHOA"
# [55] "EPHB4" "MMP1" "PAK1" "MTA1" "THBS2" "CSPG2"
# [61] "MMP17" "CD59" "DVL3" "RHOB" "COL6A3" "NOTCH2"
# [67] "BSG" "MMP11" "COL1A2" "ZYX" "RND3" "THBS1"
# [73] "RHOG" "ICAM1" "LAMA4" "DVL1" "PAK2" "ITGB2"
# [79] "COL6A1" "FGD1"
#
# $CHANG_CORE_SERUM_RESPONSE_UP
# [1] "CEP78" "LSM3" "LRRC40" "STK17A" "RPN1" "JUNB"
# [7] "NUP85" "FLNC" "HMGN2" "RPP40" "UQCR10" "AIMP2"
# [13] "CHEK1" "VTA1" "EXOSC8" "CENPO" "PNO1" "SLC16A1"
# [19] "WDR77" "UBE2J1" "NOP16" "NUDT1" "SMC2" "SLC25A5"
# [25] "NUPL1" "DLEU2" "PDAP1" "CCBL2" "COX17" "BCCIP"
# [31] "PLG" "RGS8" "SNRPC" "PLK4" "NUTF2" "LSM4"
# [37] "SMS" "EBNA1BP2" "C13orf27" "VDAC1" "PSMD14" "MYCBP"
# [43] "SMURF2" "GNG11" "F3" "IL7R" "BRIP1" "HNRNPA2B1"
# [49] "DCK" "ALKBH7" "HN1L" "MSN" "TPM1" "HYLS1"
# [55] "HAUS1" "NUP93" "SNRPE" "ITGA6" "CENPN" "C11orf24"
# [61] "GGH" "PFKP" "FARSA" "EIF2AK1" "CENPW" "TUBA4A"
# [67] "TRA2B" "UMPS" "MRTO4" "NUDT15" "PGM2" "DBNDD1"
# [73] "SNRPB" "MNAT1" "NUP35" "TCEB1" "HSPB11" "C19orf48"
# [79] "ID3" "IPO4" "FARSB" "EIF4G1" "SKA1" "MFSD11"
# [85] "PLAUR" "MARVELD2" "MCM3" "DHFR" "RNF41" "ID2"
# [91] "H2AFZ" "CDK2" "NCLN" "ZWILCH" "DYNLT1" "C16orf61"
# [97] "SLC25A40" "RHOC" "CCT5" "PDIA4" "SNRPA" "RBM14"
# [103] "PDLIM7" "PITPNC1" "TPM3" "CORO1C" "ERLIN1" "PAICS"
# [109] "TPRKB" "SKA2" "MYBL1" "SH3BP5L" "BRCA2" "SAR1A"
# [115] "POLR3K" "MRPS28" "NUP107" "TUBG1" "PNN" "FAM167A"
# [121] "RFC3" "MYL6" "MCM7" "MAGOHB" "FAM89B" "TOMM40"
# [127] "CDCA4" "MT3" "MTHFD1" "PSMD12" "MYBL2" "CKLF"
# [133] "NRIP3" "EZR" "C12orf24" "GPLD1" "SRM" "RAB3B"
# [139] "NLN" "MT1F" "TNFRSF12A" "TPI1" "HAS2" "APOO"
# [145] "FBXO41" "MRPL37" "GSTCD" "SDC1" "WDR54" "RNF138"
# [151] "APITD1" "RMND5B" "ENO1" "MAP3K8" "TMEM130" "SNX17"
# [157] "KRR1" "TAGLN" "PA2G4" "RUVBL1" "SNRPD1" "LOXL2"
# [163] "POLE2" "MAPRE1" "IMP4" "EMP2" "PSMD2" "MET"
# [169] "IFRD2" "LMNB2" "PLOD2" "NCEH1" "NME1" "STRA13"
# [175] "ACTL6A" "DLEU1" "SNRPA1" "CBX1" "LYAR" "PTPLB"
# [181] "PFN1" "CENPJ" "COTL1" "SPRYD7" "USPL1" "MRPL12"
# [187] "ADAMTS1" "GLRX3" "WSB2" "MRPS16" "DCLRE1B" "MKKS"
# [193] "C3orf26" "CPEB4" "SPAG17" "MLF1IP" "UAP1" "COQ2"
# [199] "WDHD1" "DCBLD2" "KIAA0090" "SAR1B" "PSMA7" "PSMC3"
# [205] "COPS6" "DUT" "PPIH" "PHF19" "TPM2" "MCTS1"
# [211] "EIF4EBP1" "HNRNPR"
Enjoy GSClassifier
!